Contact center analytics: The ultimate guide
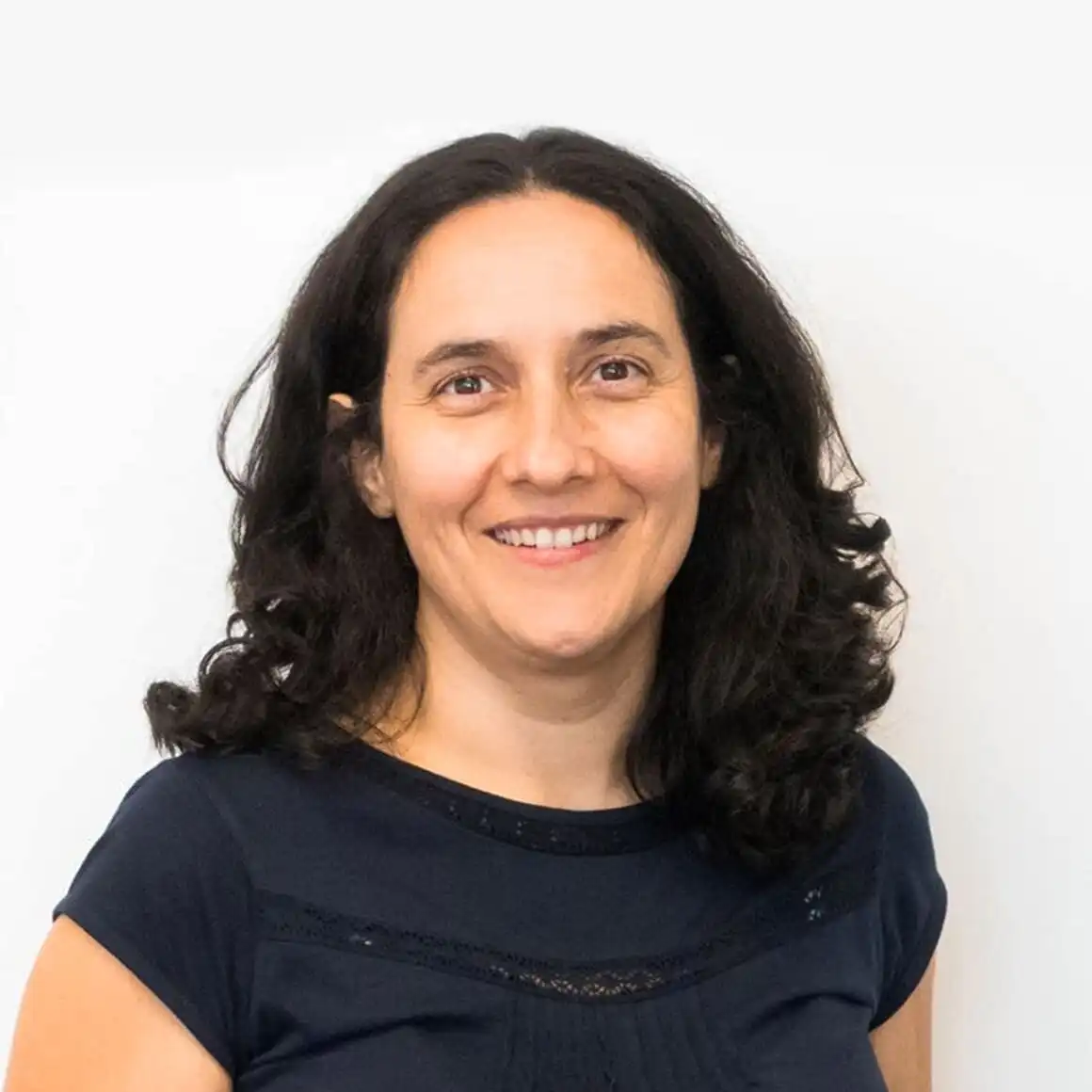
By Celia Cerdeira
0 min read
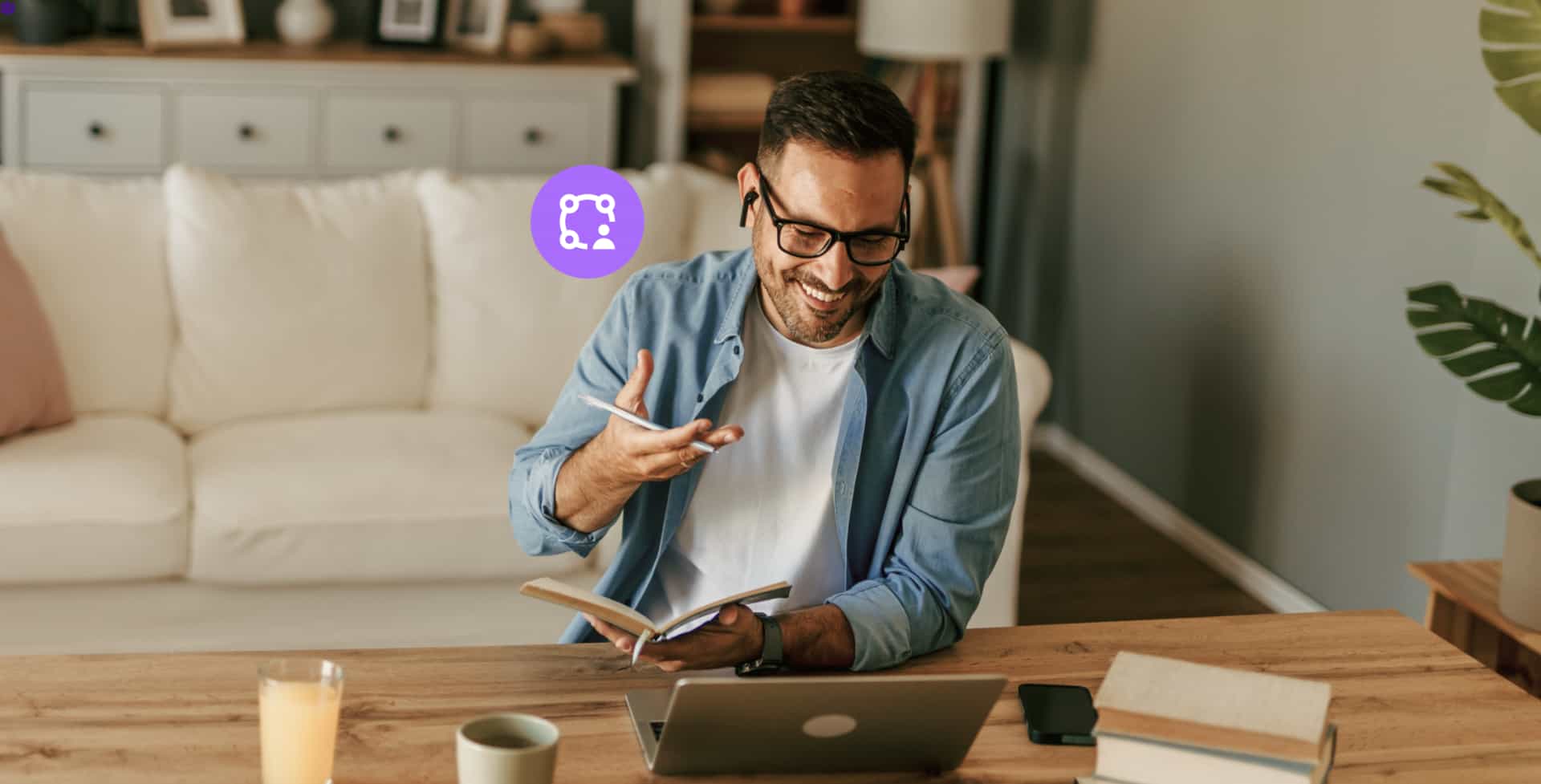
Whether calling an agent or reaching out on social media, customers expect fast, personalized, and seamless interactions. If a business can’t deliver? Customers won’t hesitate to take their loyalty elsewhere.
Contact center analytics provide a clear picture of how well a business is meeting customer needs by tracking key metrics like average handle time (AHT), first call resolution (FCR), and more. These insights help businesses fine-tune their approach, improve efficiency, and create better customer experiences.
However, manually collecting and analyzing all that data is time-consuming, and even the best teams can miss important patterns. As a result, leading organizations are turning to AI. AI-powered contact center analytics allow businesses to go beyond just tracking numbers. Instead, AI helps pinpoint problem areas and uncover trends that might otherwise go unnoticed.
In this guide, we’ll dive into why contact center analytics are essential, how they drive business success, and how AI streamlines the entire analytics process.
What are contact center analytics?
Contact center analytics is the process of collecting, measuring, and analyzing data from customer interactions to uncover insights into behavior, operational efficiency, and service quality.
Every minute an agent spends on the clock generates raw data that can be used to track business metrics and key performance indicators (KPIs). These insights help organizations evaluate performance, monitor progress toward goals, and drive improvements in customer satisfaction, agent productivity, and overall business outcomes.
However, raw data alone isn’t enough. Without the right analysis and reporting, it’s just a collection of numbers with no real direction. Contact center analytics turn this data into actionable insights, helping businesses identify strengths, address inefficiencies, and optimize CX operations.
Contact center analytics pull from a variety of sources, each offering valuable insights into interactions, agent performance, and overall operations. Here are some of the key data sources businesses rely on:
-
Customer feedback surveys. Direct feedback from customers reveals how satisfied they are and highlights areas that need improvement.
-
Agent performance data. Metrics like customer satisfaction score (CSAT) and average handle time (AHT) measure how well agents balance speed with delivering high-quality service.
-
Interaction recordings. Call, chat, and email transcripts provide a detailed look at conversations, making it easier to identify training opportunities and ensure service quality.
-
Interaction analytics. AI-powered tools analyze conversations to detect customer sentiment, uncover mood shifts throughout interactions, common issues, and emerging trends.
-
Workforce management systems. Forecasting, scheduling, and staffing data help optimize agent availability, prevent burnout, and make data-driven decisions through predictive analysis.
-
Customer relationship management (CRM) systems. Customer profiles and interaction history provide valuable context, allowing agents to personalize service and improve engagement.
Collecting this data is just the first step—knowing which analytics matter most is what truly drives improvement.
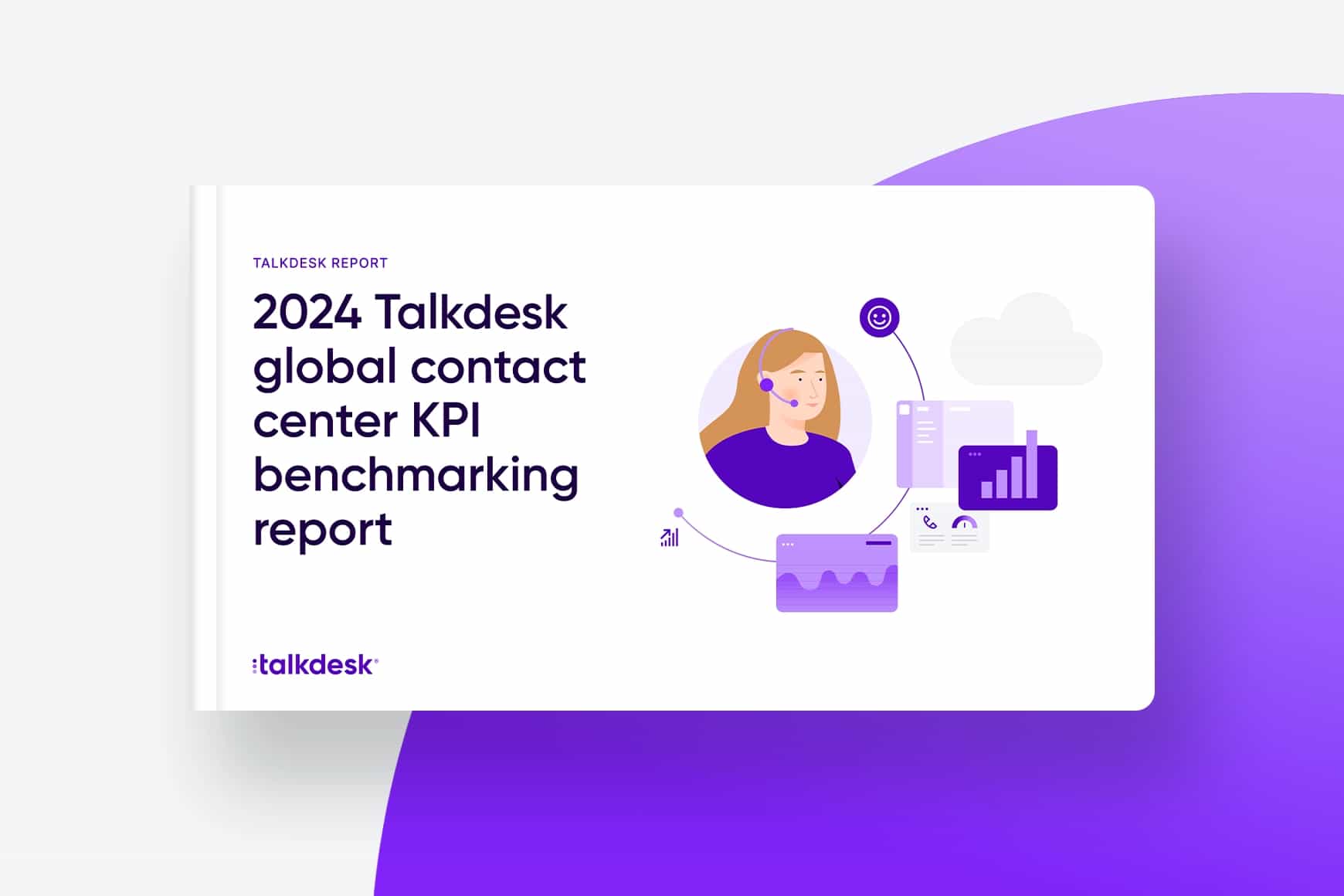
Talkdesk Report
Talkdesk 2024 KPI benchmarking report for contact centers
Get a snapshot of your contact center’s performance in the age of agentic AI, see how it compares to industry peers, and discover tips for optimization.
Which contact center analytics should an organization track?
No matter the size or industry, every organization should track key performance indicators (KPIs) to measure and improve the customer experience. Take a look at some of the most important contact center analytics that organizations should track:
-
Customer satisfaction score (CSAT). Measures how satisfied customers are with their interactions, typically collected through post-call surveys.
-
Customer effort score (CES). Evaluates how easy or difficult it was for customers to resolve their issues, highlighting potential friction points in the support process.
-
Net promoter score (NPS). Measures customer loyalty by asking how likely they are to recommend a company to others.
-
First contact resolution (FCR) rate. Tracks how often customer issues are resolved in a single interaction, reducing the need for follow-ups and improving satisfaction.
-
Customer lifetime value (CLV). Estimates the total revenue a business can expect from a single customer throughout their relationship, helping assess long-term customer impact.
-
Service level. Measures the percentage of customer inquiries answered within a set timeframe, reflecting the efficiency of a contact center.
-
Response rate. Tracks how quickly and consistently agents respond to customer inquiries across different channels.
Contact center analytics cover a wide range of metrics, helping organizations track everything from customer sentiment to agent performance and overall service efficiency. Next, we’ll break down the key types of contact center analytics and how each one contributes to a stronger, more customer-centric operation.
What are the types of contact center analytics?
Contact center analytics can be grouped into several key categories that help businesses make data-driven decisions and enhance the customer experience.
Interaction analytics analyze customer communications
Every customer interaction—whether it’s a phone call, email, chat, or social media message—tells a story. Interaction analytics help businesses make sense of these conversations by uncovering patterns in customer behavior, preferences, and pain points. With these insights, organizations can fine-tune their approach, improve communication, and proactively solve problems before they escalate.
AI has completely changed the game when it comes to interaction analytics. Instead of relying on manual call reviews or simple keyword tracking, AI-powered tools use natural language processing (NLP) and machine learning to analyze conversations in real time.
Voice analytics determine how well organizations handle phone calls.
Voice analytics help organizations understand how effectively they’re handling phone calls, still one of the most critical channels for customer service. The question isn’t just what customers are saying, but also how they’re saying it, and how agents respond in turn.
More specifically, voice analytics zero in on vocal cues like tone, pitch, volume, and pace to detect emotional states, such as frustration, satisfaction, or confusion, and track how these emotions shift throughout a call. These insights highlight what’s working well and where there’s room for improvement, helping businesses create smoother, more satisfying customer experiences.
Speech analytics, on the other hand, focuses specifically on what was actually said in a conversation. By analyzing transcribed audio, speech analytics can extract information from keywords, phrases, and content. It’s about decoding the words to understand intent, resolve issues faster, and coach agents on better responses.
Self-service analytics provide chatbot ease-of-use metrics.
With more customers turning to chatbots and automated self-service options for quick answers, businesses need a way to measure how well these tools are performing. Self-service analytics track key chatbot metrics, such as resolution rates, response accuracy, and customer satisfaction, to help organizations understand whether their chatbot is truly meeting customer needs or leaving them frustrated.
AI-powered self-service analytics continuously refine chatbot capabilities by detecting common customer queries, compensating for misspellings or ambiguous phrasing, and recognizing when an interaction needs to be escalated to a live agent. If customers frequently abandon a chatbot session or repeatedly ask the same question, this signals that the bot may need better training to interpret user intent.
Over time, self-service analytics help organizations fine-tune their chatbot experience, making interactions smoother, faster, and more intuitive.
Predictive analytics use AI to forecast future performance.
What if businesses could predict customer needs before they even arise? That’s exactly what predictive analytics do. Instead of just looking at past performance, AI-powered predictive analytics analyze patterns in customer behavior, call volumes, and agent performance to forecast future trends. This helps businesses prepare for busy periods, anticipate potential churn risks, and optimize staffing so they can stay ahead of customer expectations rather than scrambling to catch up.
For example, if a company notices that customer questions about a product spike right before a seasonal sale, it can proactively adjust staffing or update chatbot responses to handle the surge. Predictive analytics can also pinpoint when customers are most likely to need support, helping businesses schedule agents at the right times.
What tools help track contact center analytics?
To make the most of contact center analytics, businesses need the right tools to collect, analyze, and act on their data. With the right technology, organizations can transform raw metrics into meaningful insights. Let’s dive into a few essential tools.
Real-time dashboards provide all relevant contact center analytics in a single view.
Real-time dashboards give businesses instant visibility into key performance indicators (KPIs), making them one of the most important tools for monitoring contact center analytics. These dashboards consolidate all relevant data—customer satisfaction scores (CSAT), agent performance metrics, call volumes, and more—into a single, digestible view. This allows contact center agents to quickly identify emerging trends, spot inefficiencies, and take immediate action to resolve issues.
AI plays a crucial role in making real-time dashboards more powerful and actionable. Traditional dashboards often rely on static reports, requiring manual interpretation and delayed responses. AI-driven dashboards, on the other hand, analyze data in real time, highlight anomalies, and even offer predictive insights. For example, if call wait times suddenly spike, AI can alert supervisors and suggest adjustments, such as reassigning agents or prioritizing high-value customers.
Workplace management AI transforms every agent into a company’s best agent.
Great customer service starts with empowered agents, and AI is making it easier than ever to bring out the best in every one of them. Workforce management goes beyond optimizing schedules and actively helps agents perform at their peak.
By analyzing performance trends, identifying skill gaps, and automating feedback, AI provides personalized coaching and development tailored to each agent’s strengths and opportunities.
Real-time insights enable managers to intervene early, support agents who need help, and recognize top performers. AI also predicts call volumes and adjusts staffing proactively, ensuring agents are neither overwhelmed nor underutilized. The result? Smarter staffing, better support, and agents who strive for continuous improvement.
Risk and compliance reporting protects against performance issues and insider threats.
Ensuring security and compliance in a contact center is just as important as delivering great customer service. With thousands of customer interactions happening daily, businesses must protect sensitive data, monitor agent activities, and stay ahead of potential insider threats. AI-powered risk and compliance reporting tools provide real-time insights into agent behavior, security risks, and policy adherence, helping organizations manage compliance and prevent potential breaches.
AI plays a critical role in modern workforce compliance monitoring, offering automated oversight that human supervisors alone can’t provide at scale. By continuously analyzing agent activity, risk and compliance tools can detect unusual behavior patterns—such as unauthorized access attempts, data leaks, or deviations from standard procedures—and flag them for review. These tools also help ensure adherence to industry regulations like GDPR, HIPAA, and PCI DSS, reducing the risk of costly fines and reputational damage.
Quality management improves agent performance during every interaction.
Delivering a great customer experience starts with well-trained, high-performing agents. Quality management (QM) software helps businesses monitor, evaluate, and improve agent interactions in real time so that every call, chat, or email meets company standards. AI-powered QM tools offer voice and screen recording, contextualized feedback, and a seamless coaching experience. Instead of relying on random call sampling, AI analyzes up to 100% of interactions, identifying trends, strengths, and areas where agents need additional support.
One of the most powerful AI-driven QM features is Talkdesk QM Assist, which effortlessly extracts insights from interaction transcripts, identifies customer sentiment, and leverages automated scoring. For example, if an agent struggles with resolution time or deviates from compliance protocols, AI can alert managers and suggest personalized training. This helps agents improve without disrupting their workflow, fostering continuous development and boosting customer satisfaction.
What are the benefits of contact center analytics?
By leveraging the right insights, companies can optimize workflows, enhance agent performance, and create more seamless, personalized customer interactions. Here are some of the major benefits of contact center analytics:
-
Improve agent productivity. By tracking performance metrics and offering real-time coaching, businesses can help agents work more efficiently and resolve issues faster.
-
Enhance customer satisfaction. Understanding customer needs and sentiment allows businesses to refine their approach, leading to more positive and personalized interactions.
-
Save costs. AI-powered analytics optimize staffing, reduce call handling times, and improve first-call resolution, lowering operational expenses.
-
Process customer feedback. Analyzing surveys, call transcripts, and sentiment data helps businesses identify trends and adjust their strategies to better meet customer expectations.
-
Retain more customers. By proactively addressing pain points and improving service quality, businesses can boost customer loyalty and reduce churn.
-
Strengthen compliance and security. AI-powered analytics help monitor agent activity, detect potential compliance risks, and ensure adherence to industry regulations.
-
Improve omnichannel consistency. Analytics across voice, chat, email, and social media ensure customers receive a seamless experience, no matter how they reach out.
Tracking contact center analytics to improve customer service.
To improve customer service, businesses need to do more than just collect contact center analytics; they need to use them meaningfully. That means spotting trends, identifying problem areas, and making smart, data-driven changes. Consider these actionable steps to help create a better experience for both customers and agents.
1. Identify critical contact center KPIs.
Not all data is equally valuable—businesses need to focus on the right metrics to truly understand and improve their contact center performance. Identifying key performance indicators (KPIs) helps measure both efficiency and customer experience, giving companies a clear picture of where they’re excelling and where there’s room for improvement.
The best approach is to track a mix of quantitative and qualitative KPIs. For example, net promoter score (NPS), customer effort score (CES), and customer satisfaction score (CSAT) reveal how customers feel about their experiences, while metrics like average handle time (AHT), first call resolution (FCR), and call transfer rates shed light on operational efficiency.
2a. Assess contact center agent performance over time.
Tracking agent performance isn’t just about counting the number of calls handled or measuring how quickly they’re completed; it’s about evaluating the quality of interactions and their impact on the customer experience. Contact center agents play a crucial role in shaping customer satisfaction, so businesses need to continuously assess how well they’re resolving issues, handling complex inquiries, and maintaining positive interactions.
By leveraging contact center analytics software, businesses can get a well-rounded view of each agent’s performance over time. AI-powered tools help track key factors like problem-solving ability, customer sentiment, and feedback scores, providing a deeper understanding of an agent’s strengths and areas for improvement.
2b. Assess customer experience analytics.
Building great customer experiences is the result of continuously analyzing and improving interactions based on real data. AI-powered analytics make it easier than ever to measure the customer journey across different touchpoints, from phone calls and chat interactions to self-service tools and social media engagement.
By tracking customer feedback and behavioral trends, companies can pinpoint areas that need improvement and address issues before they impact customer satisfaction.
3. Track progress toward contact center analytics benchmarks.
Setting contact center analytics benchmarks is essential, but the real impact comes from continuously tracking progress toward those goals. If first-call resolution rates are improving, what changes contributed to that success? If customer satisfaction scores are slipping, what factors might be affecting the experience?
Businesses need to measure how well they’re performing against their key performance indicators (KPIs) and identify whether they’re improving, staying stagnant, or falling behind. Regularly assessing these benchmarks helps companies spot trends and make data-driven decisions that drive long-term success.
Finding the right contact center analytics solution for your organization.
Tracking contact center analytics is only half the battle; making sense of the data and taking meaningful action is the other. AI is at the heart of this process, automating data collection, spotting trends, and even predicting future challenges so organizations can stay ahead of customer expectations.
For instance, Taulia, a leading provider of working capital solutions, needed better visibility to track agent performance. Without clear insights into agent activity, they sought an AI-powered, cloud-based contact center that could provide real-time analytics and reporting. With Talkdesk, Taulia gained access to live dashboards that highlighted key performance metrics and identified coverage gaps. As a result, they were able to double their answer rate.
Additionally, the British Columbia Lottery Corporation (BCLC) turned to Talkdesk AI-powered tools to enhance both agent performance and customer experience. By leveraging speech analytics and sentiment analysis, BCLC gained deeper insights into customer interactions, allowing them to optimize workflows. The results? Decreased average hold time to 24.7 seconds, lowered handle time to 210.7 seconds, and reduced their abandonment rate to 12%.
Ready to unlock the full potential of your contact center with AI? Optimize contact center performance with Talkdesk Customer Experience Analytics and enhance efficiency with Talkdesk AI contact center software.
Contact center analytics FAQs.
Discover answers to common questions about AI-powered contact center analytics.
Contact center analytics refers to the process of gathering and analyzing data to uncover useful insights about your customers’ experience and call center productivity. AI-powered tools go beyond basic reporting by detecting patterns, analyzing sentiment, and predicting trends, enabling organizations to proactively improve customer experiences and streamline workflows.
The most important contact center analytics to track include customer satisfaction metrics like customer satisfaction score (CSAT), net promoter score (NPS), and customer effort score (CES), as well as operational metrics such as first-call resolution (FCR), average handle time (AHT), and service level.
Contact center analytics are essential for understanding customer behavior, agent performance, and operational efficiency in real time. AI detects patterns, predicts trends, and automates insights, allowing businesses to spot issues before they escalate, optimize workflows, and enhance customer experiences.
Companies need AI-powered analytics tools to effectively track contact center performance, customer interactions, and agent productivity. Solutions like real-time dashboards, speech and text analytics, workforce management AI, and predictive analytics provide actionable insights that help businesses improve customer experiences.
Contact center analytics offer valuable insights into customer interactions, preferences, and pain points. By analyzing customer data, businesses can identify recurring issues, proactively resolve problems, and personalize service to better meet customer needs. These insights drive smarter decisions, improving customer satisfaction.
AI automates the collection and analysis of vast amounts of data, making the process faster and more accurate. AI-powered analytics tools can analyze speech and text in real time, providing instant insights into customer sentiment and agent performance. They can also predict trends and patterns, helping you anticipate customer needs and adjust your strategies accordingly.
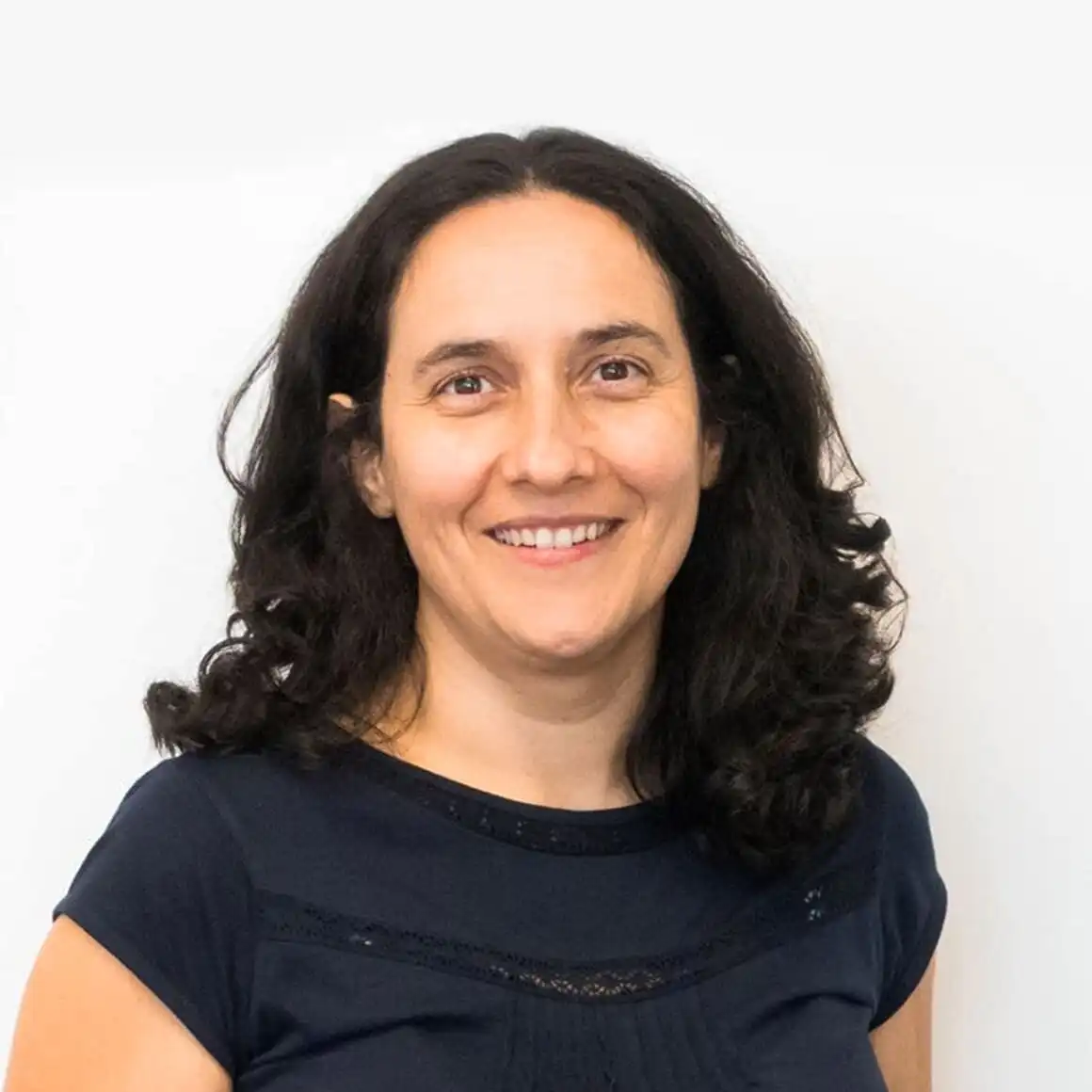