Eliminating AI bias from bank decision-making
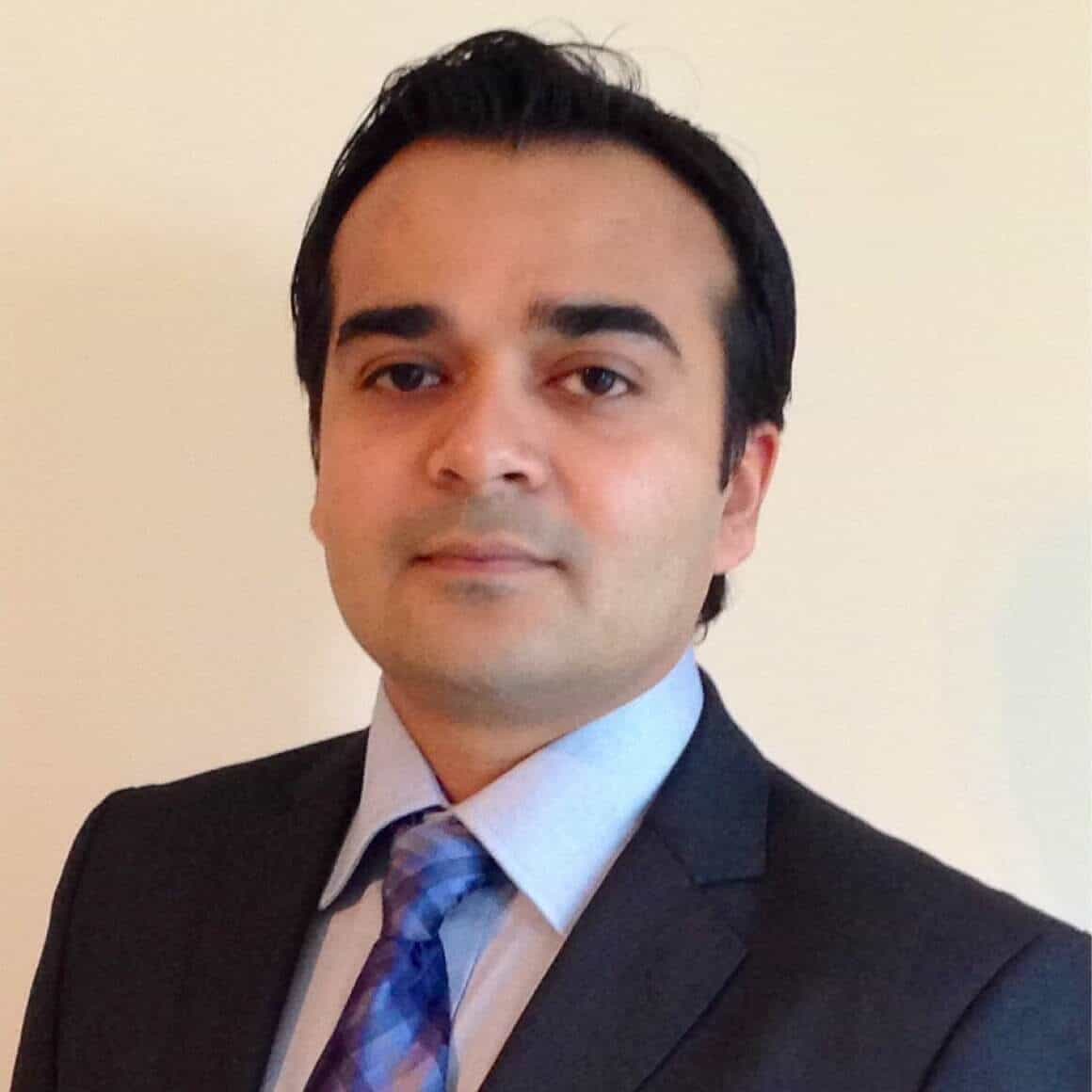
By Rahul Kumar
0 min read
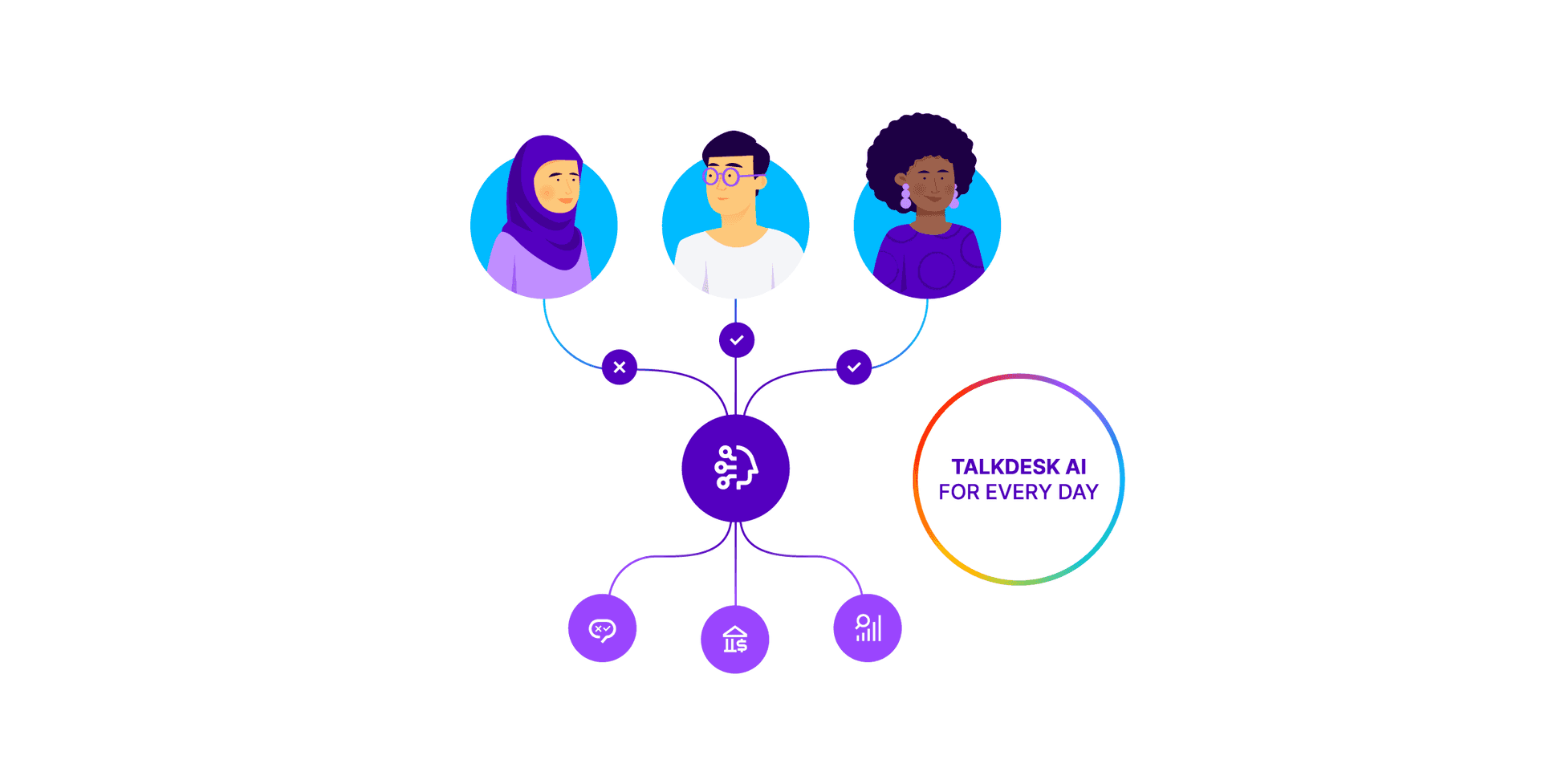
Comprehensive policies and processes to reduce the possibility of flawed algorithms can reduce the risk of discriminating against customers.
The banking industry’s use of machine learning (ML) and artificial intelligence (AI) is expected to skyrocket in the next few years as it strives to deliver hyper-personalized client experiences, improve operational efficiencies and expand product and service offerings.
In a recent survey of global banking IT executives, 81% agreed that “unlocking value from AI will distinguish winners from losers,” while 85% said they have a “clear strategy” for new products and services using AI.
ML and AI have the potential to provide numerous benefits for banks and credit unions, including:
- Delivering relevant, contextual insights to improve the overall financial well-being of clients.
- Enabling banks to offer convenient and coordinated digital customer experiences in the channel of their preference.
- Driving efficiency and productivity in assisted channels, leading to higher employee satisfaction.
- Detecting and mitigating fraud proactively, and ensuring compliance.
While it’s tempting to assume that intelligent machines and algorithms are free of human flaws, the truth is that humans can inadvertently inject their own conscious and unconscious biases into the algorithms that drive AI/ML learning and decision-making. A result can be discrimination against some clients by deciding whether to approve mortgages and other large loans based on flawed training data. A 2021 Federal Reserve study shows the algorithmic systems used by some mortgage underwriters denied applications from minority borrowers at a higher rate than non-minorities.
Rohit Chopra, director of the Consumer Financial Protection Bureau, has warned about AI bias leading to “digital redlining” and “robot discrimination,” and vowed the bureau would take a “deeper look at how lenders use artificial intelligence or algorithmic decision tools.” Two House committee chairs have asked regulators to ensure safeguards against bias against low- and middle-income families and people of color applying for loans.
Below are specific steps financial institutions can take to minimize, if not eliminate, bias in their AI/ML models.
Commit to diversity within your data and decision science teams. Our societies are more multicultural today than ever before, and financial institutions should take advantage of that to build a diverse workforce. A diverse team is a safeguard against biases bred by a homogenous workforce.
Create a multidisciplinary team for AI initiatives that consists of not just developers and business managers, but also HR and legal professionals.
Ensure strong governance to minimize the risk of bias and discrimination. Create multidisciplinary internal teams or contract outside parties to audit AI models and analyze data. Establish a policy of full transparency regarding the process of developing both AI algorithms and metrics for measuring bias, and keep up with regulations and best practices.
Use reverse-engineering to gain visibility into black-box algorithms. (Note: This may not be possible for “strong black boxes” that can’t be analyzed by reverse-engineering.)
Build diverse data sets and harness unstructured data from internal and external sources to carve a pathway to inclusivity. Check constantly for skewed or biased data, particularly during data ingestion but also at several stages of model development.
Monitor AI/ML models for data drift and concept drift, and teach model users how to monitor for issues. Scan training and testing data to determine if protected characteristics and/or attributes are underrepresented. Retrain models when issues are detected.
Keep a “human in the loop” to not only make AI a welcomed “everyday helper,” but also to continuously train it to learn new things, serve more customers and break down traditional communication barriers at scale. This will enable a bank’s own team members to promote inclusivity and make every client feel heard and respected.
Bias in AI algorithms can be difficult to detect, and often visibility is gained only after the damage has been done. Unless banking institutions adopt comprehensive policies and processes to reduce the possibility of AI bias, they run the risk of discriminating against customers. This could lead to lawsuits, brand damage and financial penalties.
As Maya Angelou said, “I’ve learned that people will forget what you said, people will forget what you did, but people will never forget how you made them feel.” A bank’s ability to prevent and remove bias in its AI/ML models can go a long way toward determining how well it will succeed serving clients and as an organization.
This blog was first published at BAI.
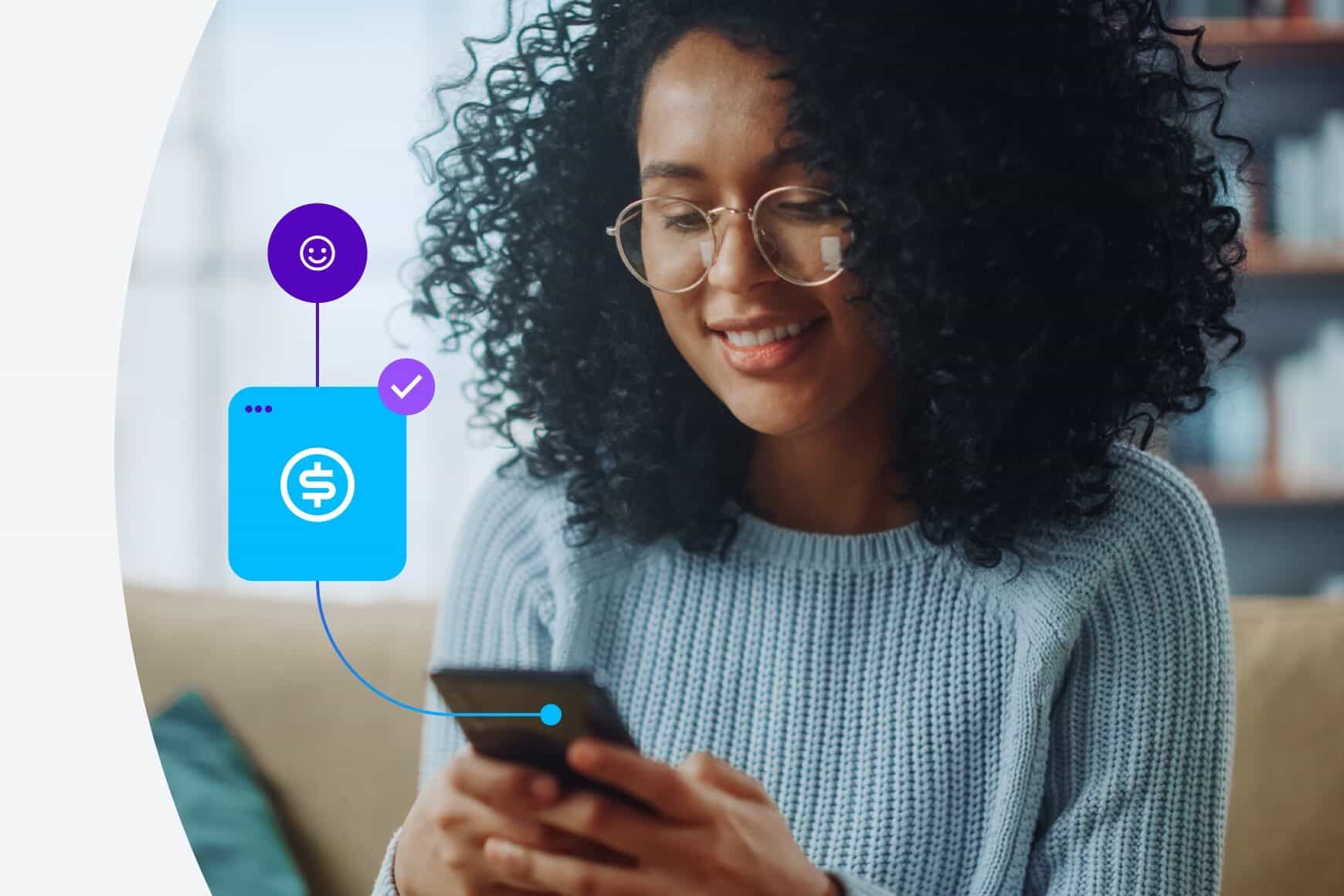
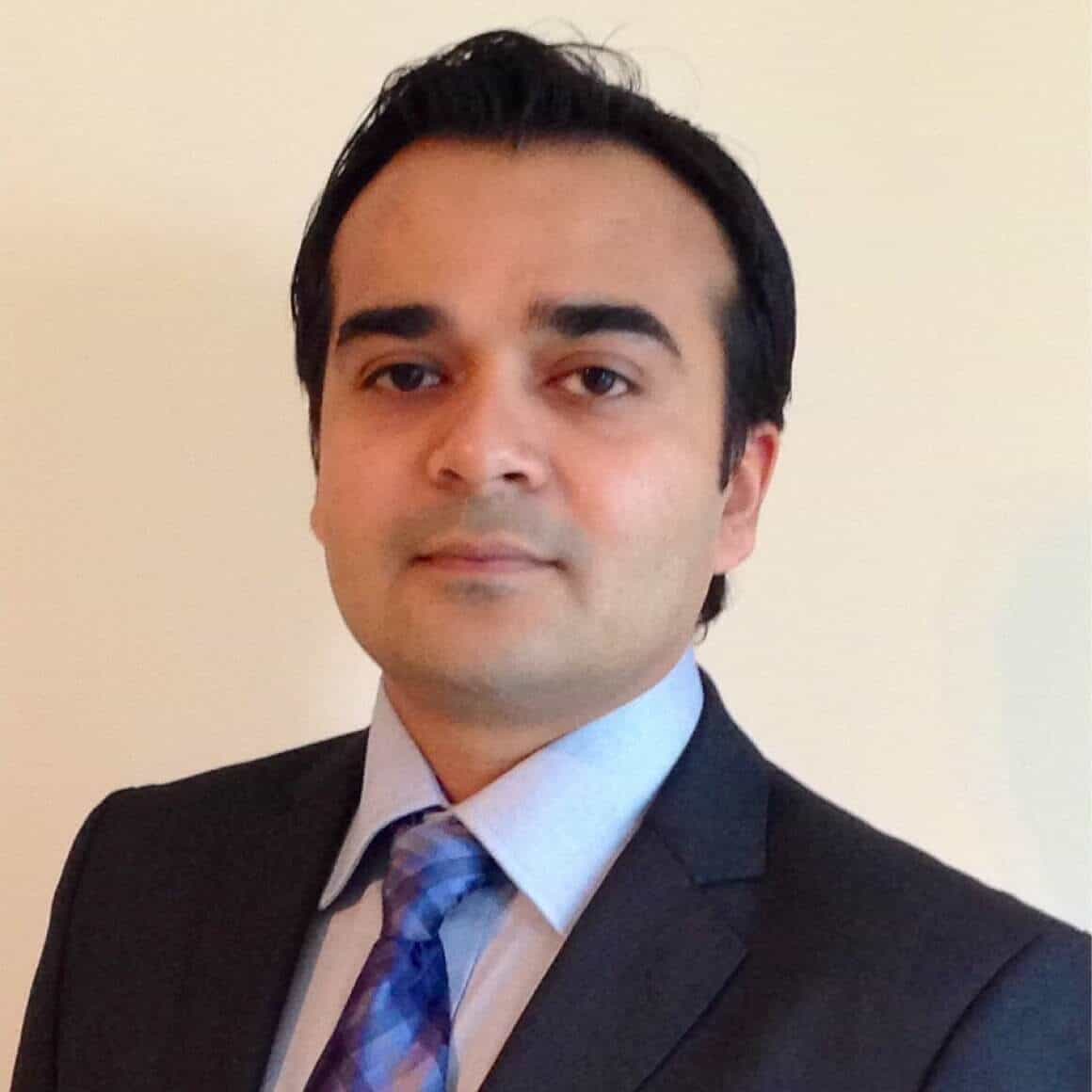